Making decisions is tough in today's fast-paced world. Because technology is constantly improving and so much data exists, organizations need to quickly analyze information and weigh many different factors. To deal with this complexity, many are using the powerful combination of AI and simulation modeling to make better decisions, leading to both innovation and improved efficiency.
Understanding AI and Simulation Modeling
Artificial Intelligence (AI) is about creating computer systems that can perform tasks requiring human intelligence. This includes tasks like reasoning, learning, and understanding language. On the other hand, simulation modeling involves creating virtual representations of real-world processes or systems. It helps users explore potential outcomes by adjusting various inputs and variables.
By merging these two technologies, organizations can build more precise models and forecasts, which leads to better strategic planning and operational effectiveness.
The Role of AI in Enhancing Simulation Modeling
AI significantly boosts the capabilities of simulation modeling. Traditional simulation often relies on static data and fixed algorithms, which can be restrictive. Including AI enables these models to be more adaptive and intelligent.
Data-Driven Insights
AI algorithms shine when it comes to processing large datasets. For example, a retail company might analyze customer behavior from millions of transactions to identify shopping trends. Feeding simulations with real-time data allows businesses to create dynamic models that more accurately reflect current conditions. A study found that organizations using AI-driven insights saw a 25% increase in operational efficiency.
Predictive Analytics
Thanks to AI, simulation models have evolved into advanced predictive tools. These models can simulate various future scenarios, allowing companies to forecast the potential impact of their decisions. For instance, a financial service company might use predictive analytics to simulate market movements and adjust investment strategies accordingly, enabling proactive planning and risk management.
The Benefits of Combining AI and Simulation Modeling
The integration of AI and simulation modeling brings a host of benefits to modern decision-making.
Improved Accuracy
Leveraging AI enhances the precision of simulation models. For example, a logistics company using AI-driven simulations can predict delivery times with 95% accuracy, significantly reducing the risk of delays that impact customer satisfaction.
Faster Decision-Making
In a fast-moving environment, quick decisions can be critical. AI automates data analysis and scenario generation in simulation models, cutting down on the time needed for decision-making. In industries like finance, this can mean executing trades in just a few seconds rather than minutes.
Enhanced Resource Allocation
AI facilitates more sophisticated analysis regarding resource allocation. For example, hospitals can use simulation models to evaluate the effects of various staffing strategies, allowing them to optimize their personnel levels and save up to 30% on labor costs.
Real-World Applications of AI and Simulation Modeling
The positive impact of combining AI and simulation modeling is evident in various sectors. Here are a few notable examples:
Healthcare
In healthcare, AI-enhanced simulation modeling is transforming patient care. Hospitals can model patient flow to anticipate needs and allocate resources accordingly. A study showed that using these techniques improved patient wait times by 20%, resulting in better care and increased satisfaction.
Manufacturing
Manufacturers apply AI-backed simulation models to streamline production. For instance, a company might simulate different machine setups to determine the most efficient production line configuration. This approach can lead to a 15% reduction in operational costs by minimizing downtime.
Transportation
Transportation networks are another area where AI and simulation modeling shine. Companies simulate traffic patterns to optimize routes and improve delivery times. For instance, a logistics firm employing these methods saw an increase in on-time deliveries by 40%.
Challenges and Considerations
Despite the benefits, incorporating AI and simulation modeling comes with its own set of challenges.
Data Quality
The success of AI-driven simulation models strongly depends on the quality of input data. Inaccurate or incomplete data can lead to flawed results. For instance, a company using outdated customer data might misjudge market trends, resulting in poor product launches.
Complexity of Models
Building and maintaining complex AI-enhanced models can be resource-intensive. Organizations need to consider if the prospective benefits outweigh the time and investment required. Many companies find it useful to start small and scale up as they gain expertise.
Ethical Considerations
Data privacy and algorithmic bias are crucial concerns in AI technologies. Companies must take steps to ensure their models treat all users fairly and respectfully. Regular audits and transparency in algorithm development can help build trust.
Final Thoughts
The collaboration between AI and simulation modeling is reshaping the decision-making landscape for organizations today. By leveraging real-time insights and predictive capabilities, businesses can address the complexities of modern environments with increased precision and speed. However, embracing these technologies also requires awareness of the inherent challenges and ethical responsibilities.
To thrive in an uncertain world, organizations must not only adopt these advancements but also approach them thoughtfully.
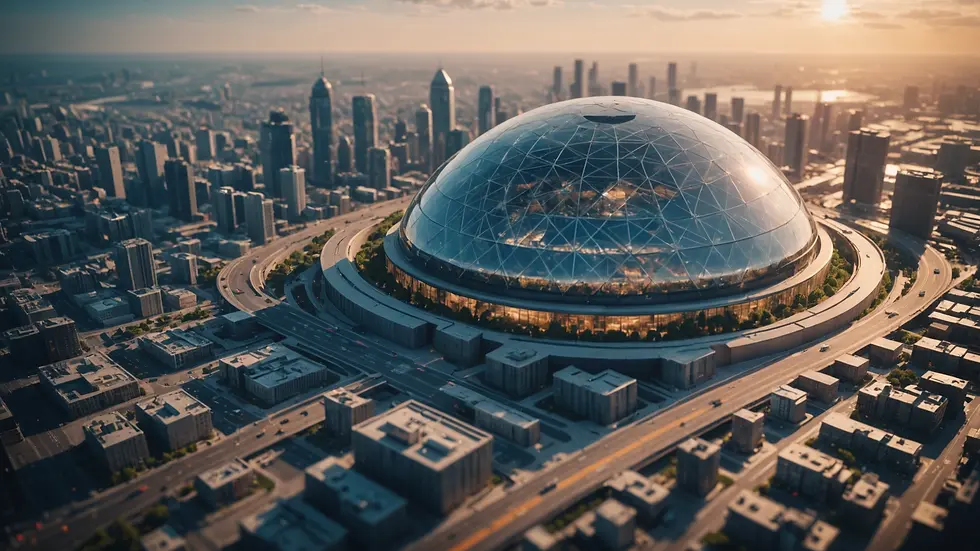
Commenti